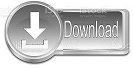
The results have been checked with a medical professional, and they successfully support clinical diagnosis. Moreover, we have achieved a very high specificity (>97%), crucial in diagnosis. Our algorithm can detect bowel sounds with an accuracy >93%. Previous works had ignored this period and instead kept brief records only during the day.

Data have been collected at night-time, which is the most interesting period from a neurogastroenterological point of view. We have developed an experimental pipeline and evaluated our results with a new dataset collected using a device with a dedicated contact microphone. It is one of the first methods of using deep learning to be widely explored. This article proposes a novel methodology for the analysis of BS using hybrid convolutional and recursive neural networks. Clinical research on BS had revealed their high potential in the non-invasive investigation of irritable bowel syndrome to study gastrointestinal motility and in a surgical setting. Accuracy of ~90% had been achieved by several teams using various analytical approaches. Attribute detectors with CNN, versus DBN-DNN model, reduce the AEER producing 10.42% relative improvements for manner of articulation and producing 13.11% relative improvements for place of articulation.Īutomated bowel sound (BS) analysis methods were already well developed by the early 2000s. The results of the work are shown in terms of average equal error rate inferring that the attribute model based on the CNN consistently outperforms the DBN-DNN model. The detectors work by detecting phonologically distinctive articulatory features of Arabic based on manner and place of articulation, which extract characteristics of the human speech production process from the speech dataset.
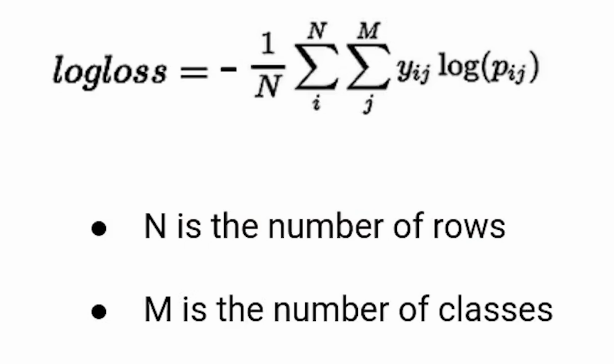
BINARY CROSS ENTROPY CODE
These attribute detectors are trained using a data-driven approach for Arabic speech data using the Buckwalter code for Arabic transcription. Two state-of-the-art deep neural networks were used for attribute speech feature detection, convolutional neural networks (CNNs) and feed-forward neural network, with pretraining using a stack of restricted Boltzmann machines (DBN-DNN).

ASAT is a new family of lattice-based speech recognition systems grounded on accurate detection of speech attributes. This approach integrates both top-down and bottom-up knowledge into the automatic speech attribute transcription (ASAT) framework. In this work, we present an alternative model to Arabic speech recognitions to boost classification accuracy of basic speech units in Industrial Internet of Things.
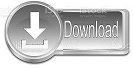